








Sigma-Lamaque project / Integra Gold Rush
Gathered as the Data Miners team, we took part in the Integra Gold Rush Challenge, organized by Integra Gold Corp.
The goal was to process 6To of data from the Sigma-Lamaque property near Val d'Or (QC, Canada) and provide the company with exploration targets as well as an innovative method to process such a large amount of data.
We tackled the problem by elaborating an integrative method to improve raw data, generate an accurate 3D geological model (using Leapfrog Geo software), extract a 3D grid with all mineral exploration vector-related data, and process the data with a Machine-Learning algorithm that identifies prospective zones.

Modified from Google Earth
Location
The Sigma and Lamaque mines are classic examples of shear-hosted, quartz-tourmaline-vein type gold deposits occuring along first-order fault zones; the Lader Lake-Cadillac fault zone in the Val d'Or district in southeastern part of the Abitibi greenstone belt.
​
​
Our Approach
- Mineral exploration is a continuously evolving industry despite its conservative reputation
- Increasing amounts of data are collected to discover deposits
- Today’s methods of treating and interpreting data are becoming obsolete.
​
Our Solution: Artificial intelligence
Machine learning (artificial intelligence) is used in all applied fields to classify, interpret and help decision making over hudge databases
​
The potential in the mining industry is tremendous (exploration, metallurgy, exploitation) and applications already exist (geological mapping, prospectivity, metallurgy).
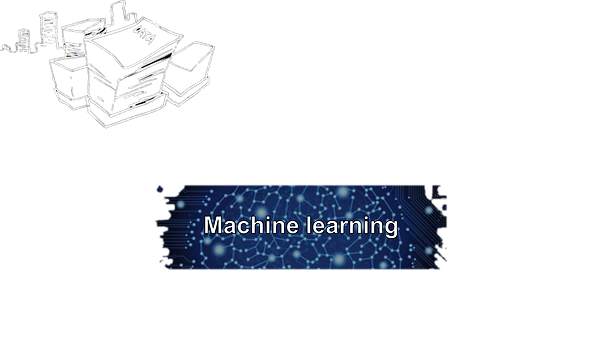
No human brain can process this amount of data!
​
This is why we generated the targets using the most recent and powerful, machine learning algorithm.
​
Machine learning is fairly simple. We first provide the algorithm with all the data.Then with our knowledge about the data, geology and mining industry tell it what we are looking for – whether low grade/high tonnage deposits or high grade/low tonnage deposits; and it will identify reliable prospective zones in only a few minutes.
Data Miners Workflow
The essence of our method is the combination of rigorous geological modeling with machine learning (artificial intelligence). The algorithm we use studies spatial relationships between numerous primary variables and generates spatially continuous predictions for potential mineralization.
The major strenghs of our workflow include: drastic reduction of human-related errors, processing of extensive datasets, classification of key variables controlling gold distribution, swift identification of reliable exploration targets, and ease of exploration model improvements with new data input.
